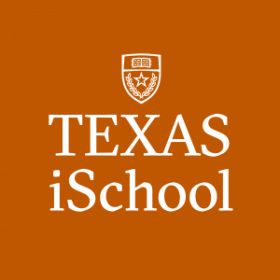
Talk 1: ProtoTEx - Explaining Model Decisions with Prototype Tensors
Abstract: We present ProtoTEx, a novel white-box NLP classification architecture based on prototype networks (Li et al., 2018). ProtoTEx faithfully explains model decisions based on prototype tensors that encode latent clusters of training examples. At inference time, classification decisions are based on the distances between the input text and the prototype tensors, explained via the training examples most similar to the most influential prototypes. We also describe a novel interleaved training algorithm that effectively handles classes characterized by ProtoTEx indicative features. On a propaganda detection task, ProtoTEx accuracy matches BART-large and exceeds BERTlarge with the added benefit of providing faithful explanations. A user study also shows that prototype-based explanations help non-experts to better recognize propaganda in online news.
Bio: Anubrata Das is a Ph.D. student at the School of Information at the University of Texas at Austin, co-advised by Dr. Matt Lease and Dr. Jessy Li (Linguistics). He is a part of the Information Retrieval and Crowdsourcing Lab and associated with the UT Austin NLP Group. Das is interested in the intersection of Natural Language Processing and Human-Computer Interaction. His research focus is on building algorithms and tools that facilitate human-AI partnerships. Das investigates the following broad questions: How can explainable AI help in the human decision-making process, especially when the AI is not perfect? How do we collect datasets, build explainable AI systems and evaluate them? His work also extends explainable models for moderating harmful content online which includes misinformation, hate speech, disruptive or obscene content, and unfairness in information access algorithms.
Talk 2: Designing for Safe, Fun and Informative Online Cross-Partisan Interactions
Abstract: The past decade in the US has been one of the most politically polarizing in recent memory. Increasingly, ordinary Democrats and Republicans fundamentally dislike and distrust each other, even when they agree on policy issues. Most Americans report believing that the opposing party is a serious threat to country. In this context, my research aims to reduce hostile interactions and attitudes towards ordinary Democrats and Republicans by leveraging nonpolitical online spaces that cut through the partisan faultlines in uniquely engaging ways. In this talk, I will touch upon on three studies, (i) a large-scale computational analysis of political discussions in nonpolitical online communities, (ii) an interview study of Reddit users who talk politics with opposing partisans and (iii) a fun party game aimed to reduce partisan hostility, to demonstrate the potential of using nonpolitical context to facilitate quality online cross-partisan interactions that account for and mitigate the heightened levels of partisan animosity we observe today.
Bio: Ashwin Rajadesingan (PhD, Information, University of Michigan) is a computational social scientist and design researcher studying how people engage with politics online. He is interested in how online spaces can foster spirited, engaging political discussions and contribute to building a vibrant deliberative democracy. In his research, he combines large-scale computational methods with qualitative interviews to (re)design online spaces that facilitate quality online cross-partisan interactions. His work has won multiple best paper awards and has been published in the proceedings for AAAI and ACM conferences such as ICWSM, CSCW, WSDM and SMSociety. He was awarded a Facebook Fellowship in Computational Social Science in 2020.